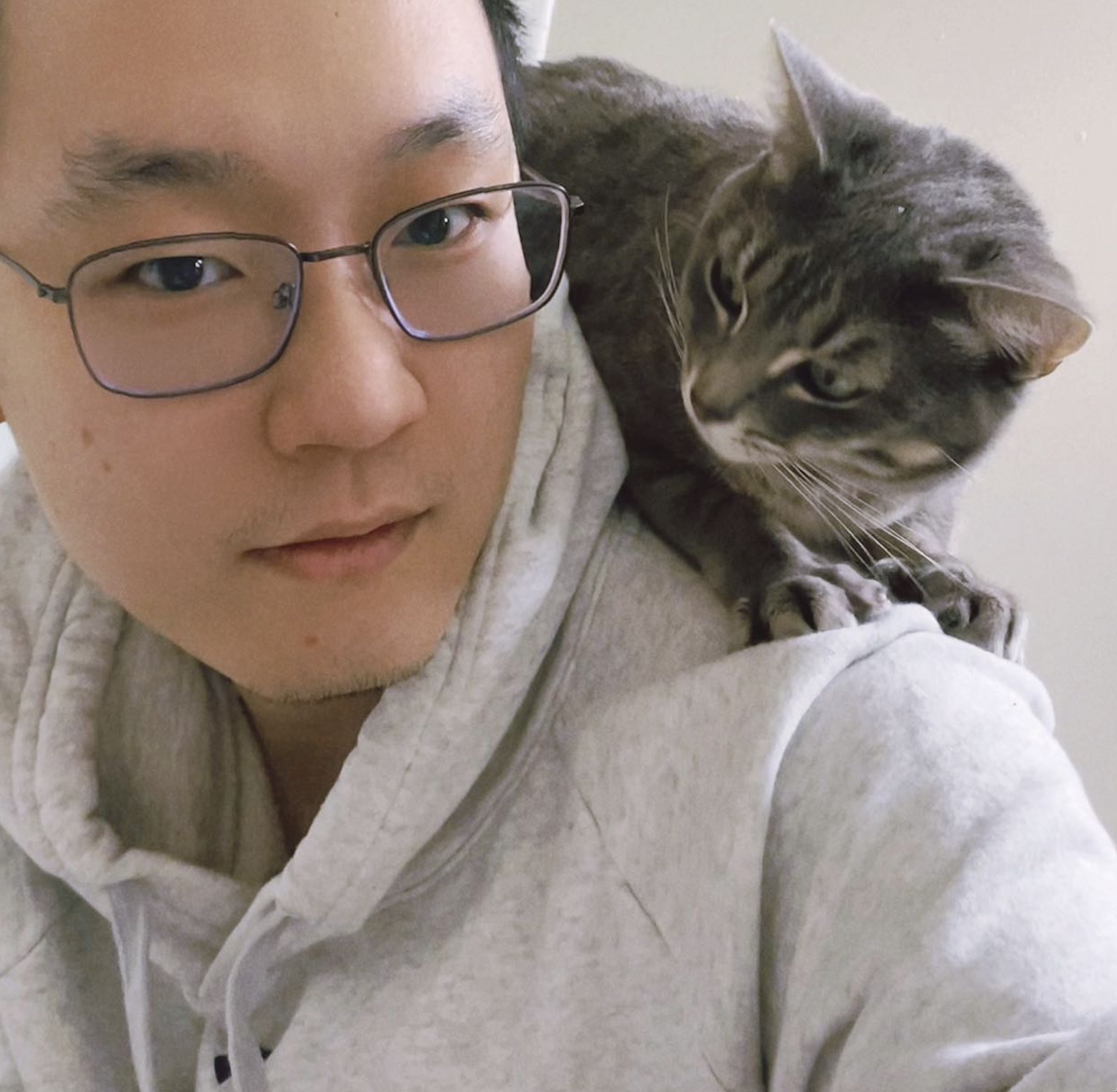
Ph.D. Student, Yale University
chen.liu.cl2482 at yale.edu
New Haven, CT & Mountain View, CA.
Google Scholar
Twitter
LinkedIn
GitHub
Acknowledgements Many thanks to Zhuang Liu for kindly providing this website template, which was adapted from Zhe Cao's website.
Chen Liu
I am looking for a research internship (Summer 2025). Please let me know if you have opportunities in spatial-temporal modeling, multimodal learning, self-supervised learning, manifold learning, AI in healthcare, or related fields.
I am a Ph.D. student in computer science at Yale University (2022~) advised by Prof. Smita Krishnaswamy. I received my M.S. from Columbia University (2018~2020), and I did my undergraduate studies at a liberal arts college, Bucknell University (2014~2018).
Research Areas At the moment, my research briefly touches the theory and application of machine learning. On the theory side, I help neural networks learn better representations in the latent space, and one of my most recent work focus on modeling spatial-temporal dynamics in irregularly-sampled image series. On the application side, I extend my research to medical imaging, proteomics and other biomedical data.
During the second half of my Ph.D., I would like to endeavor a more specialized direction, which is modeling the spatial-temporal dynamics of complex systems, such as the disease progression revealed in medical images acquired over time.
Experience Prior to my Ph.D., I first served as a full-time research assistant at Columbia University Medical Center (2020) in a medical imaging lab. The next year, I went to the industry and joined a startup company Matic (2021) and developed SLAM algorithms for housekeeping robots. Following that, I worked as a Senior Research Scientist at GE Healthcare (2021~2022), on deep learning in medical imaging applications, where I co-invented 2 patents.
News
[07/2024] I wrote a tool to generate your Google Scholar Citation World Map. [PDF] [Code]
[06/2024] My first project during my Ph.D. was accepted to MICCAI 2024. [Paper] [PDF] [Code] [MICCAI] [Poster]
[08/2022] I started my Ph.D. journey at Krishnaswamy Lab, Yale University.
[06/2022] I was recognized as an Outstanding Reviewer at ICML 2022.
Selected Recent Publications (* equal contribution)
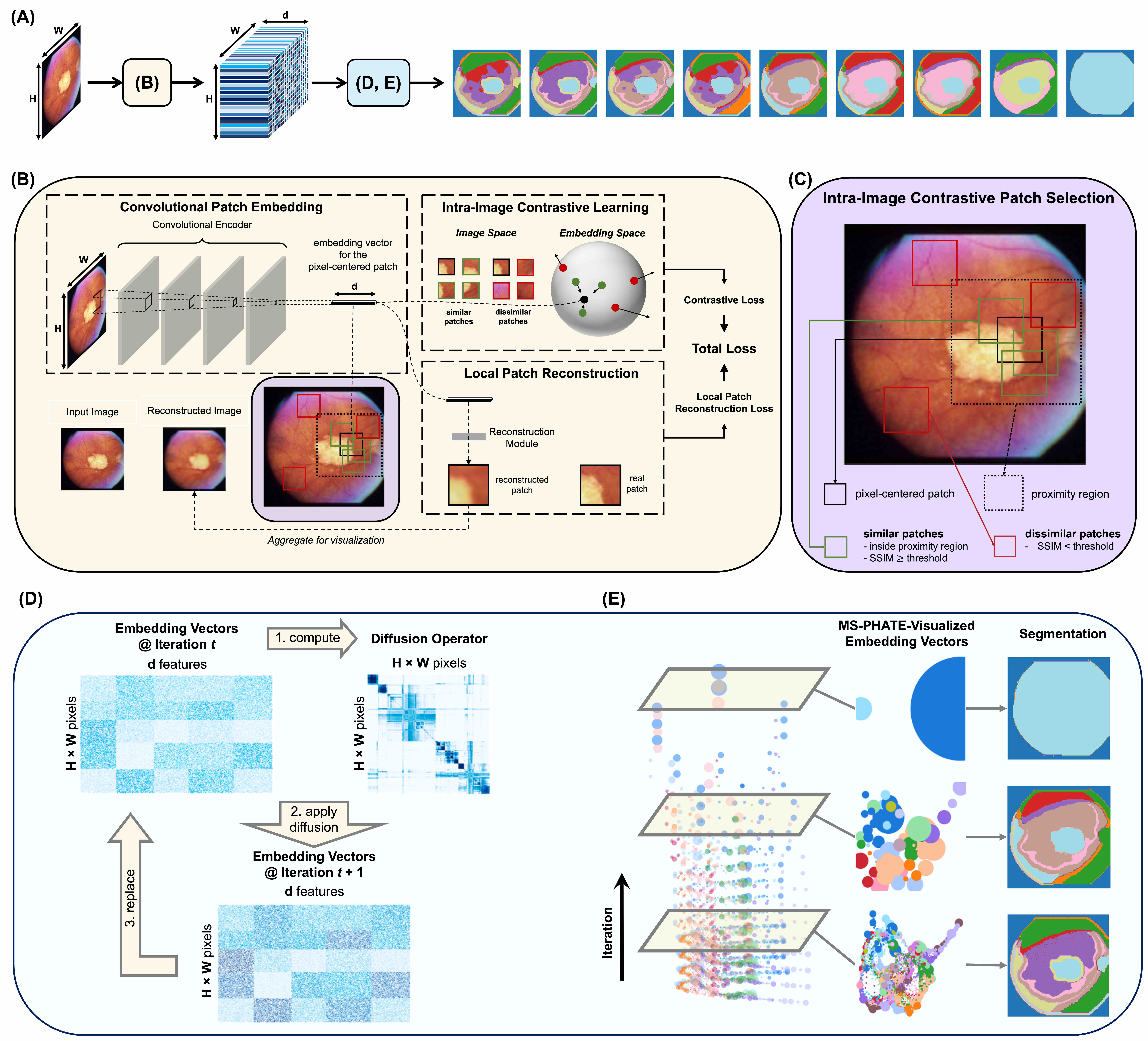
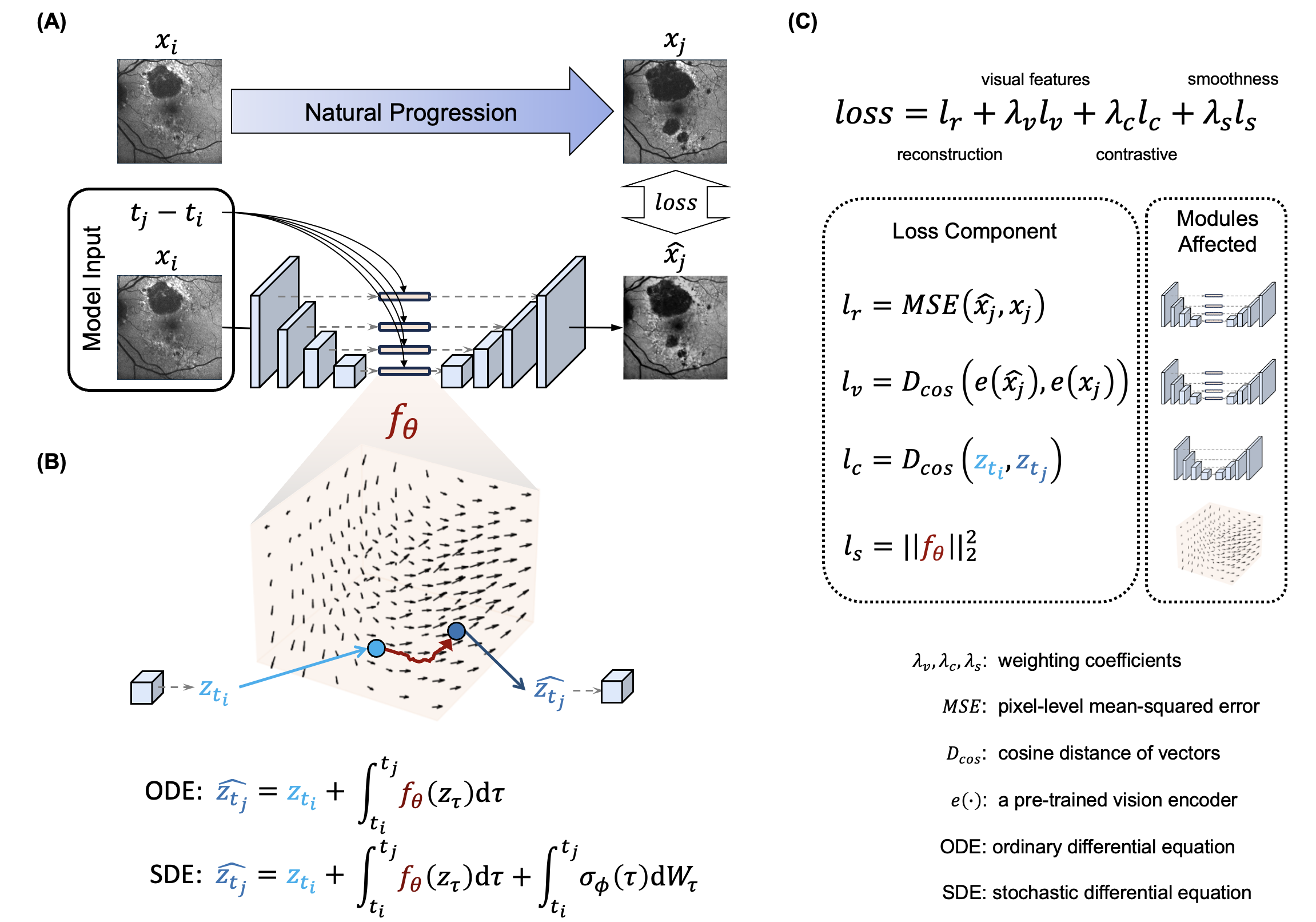
⏳ ImageFlowNet: Forecasting Multiscale Image-Level Trajectories of Disease Progression with Irregularly-Sampled Longitudinal Medical Images
Chen Liu*, Ke Xu*, Liangbo L. Shen, Guillaume Huguet, Zilong Wang, Alexander Tong, Danilo Bzdok, Jay Stewart, Jay C. Wang, Lucian V. Del Priore, Smita Krishnaswamy
arXiv 2024
Conference Papers (* equal contribution)
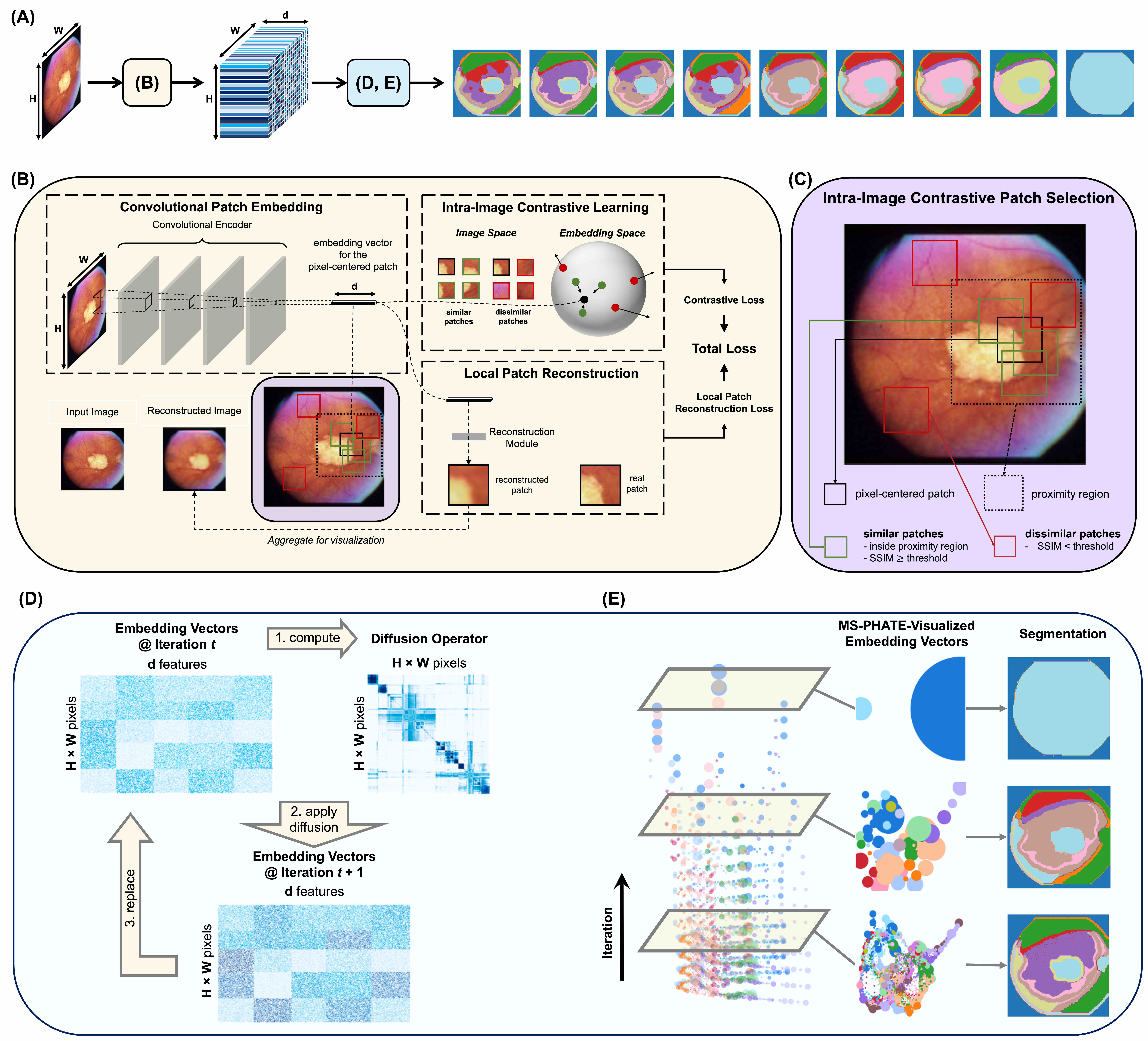
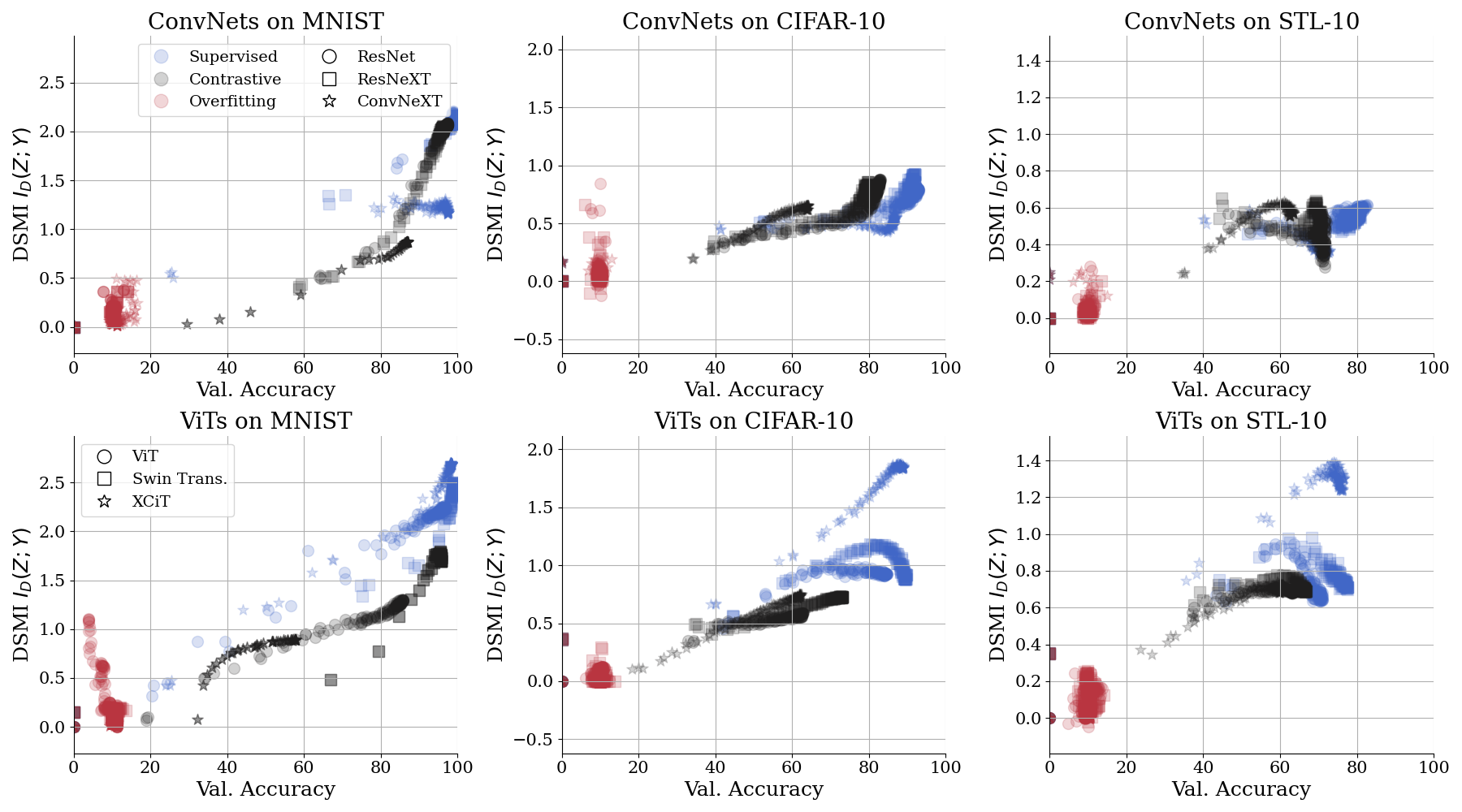
🎲 Assessing Neural Network Representations During Training Using Noise-Resilient Diffusion Spectral Entropy
Danqi Liao*, Chen Liu*, Benjamin W. Christensen, Alexander Tong, Guillaume Huguet, Guy Wolf, Maximilian Nickel, Ian Adelstein, Smita Krishnaswamy
ICML 2023 TAG-ML Workshop & IEEE CISS 2024
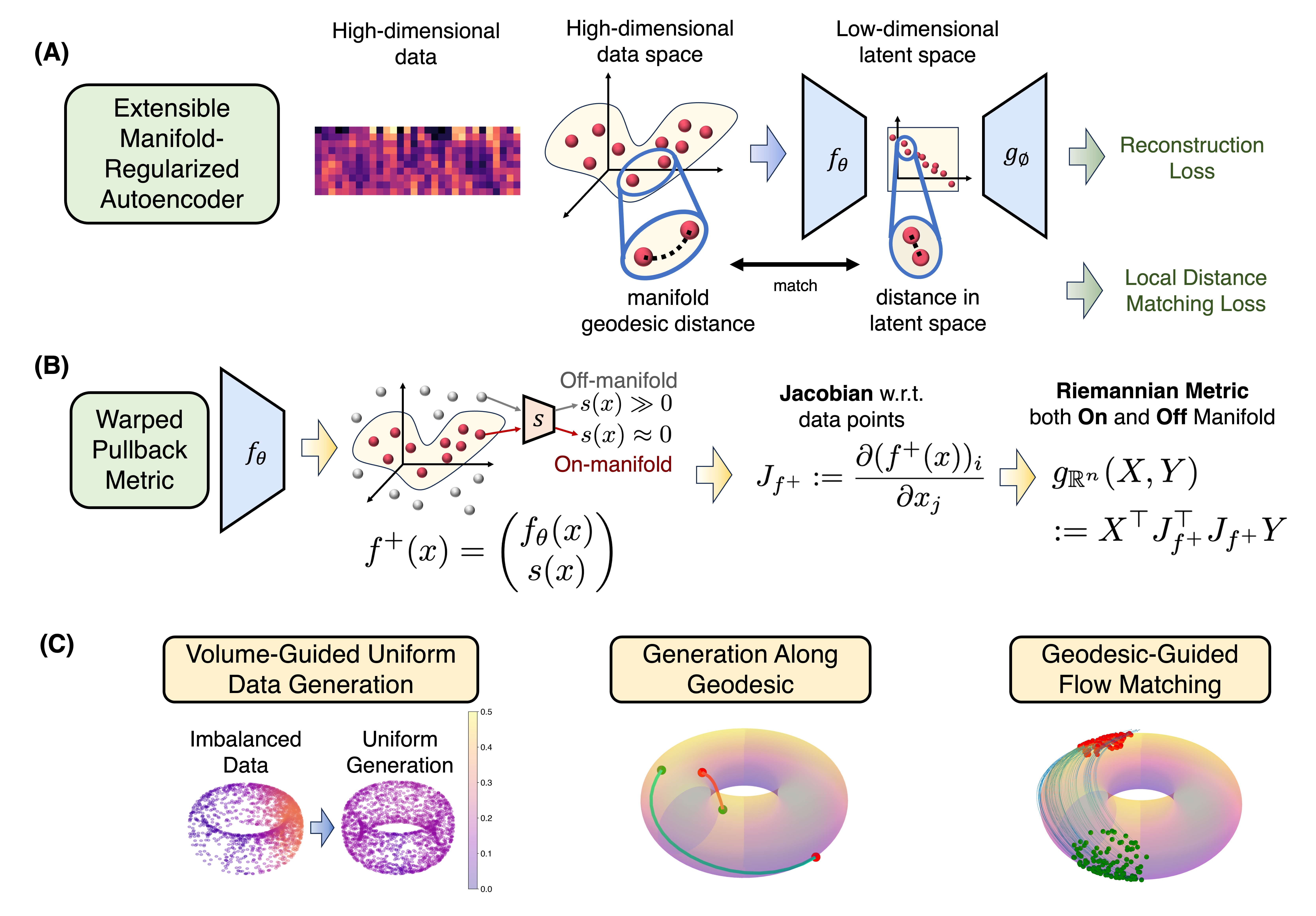
🌐 Geometry-Aware Generative Autoencoders for Warped Riemannian Metric Learning and Generative Modeling on Data Manifolds
Xingzhi Sun*, Danqi Liao*, Kincaid MacDonald*, Yanlei Zhang, Chen Liu, Guillaume Huguet, Guy Wolf, Ian Adelstein, Tim GJ Rudner, Smita Krishnaswamy
ICML 2024 GRaM Workshop & under review at a conference
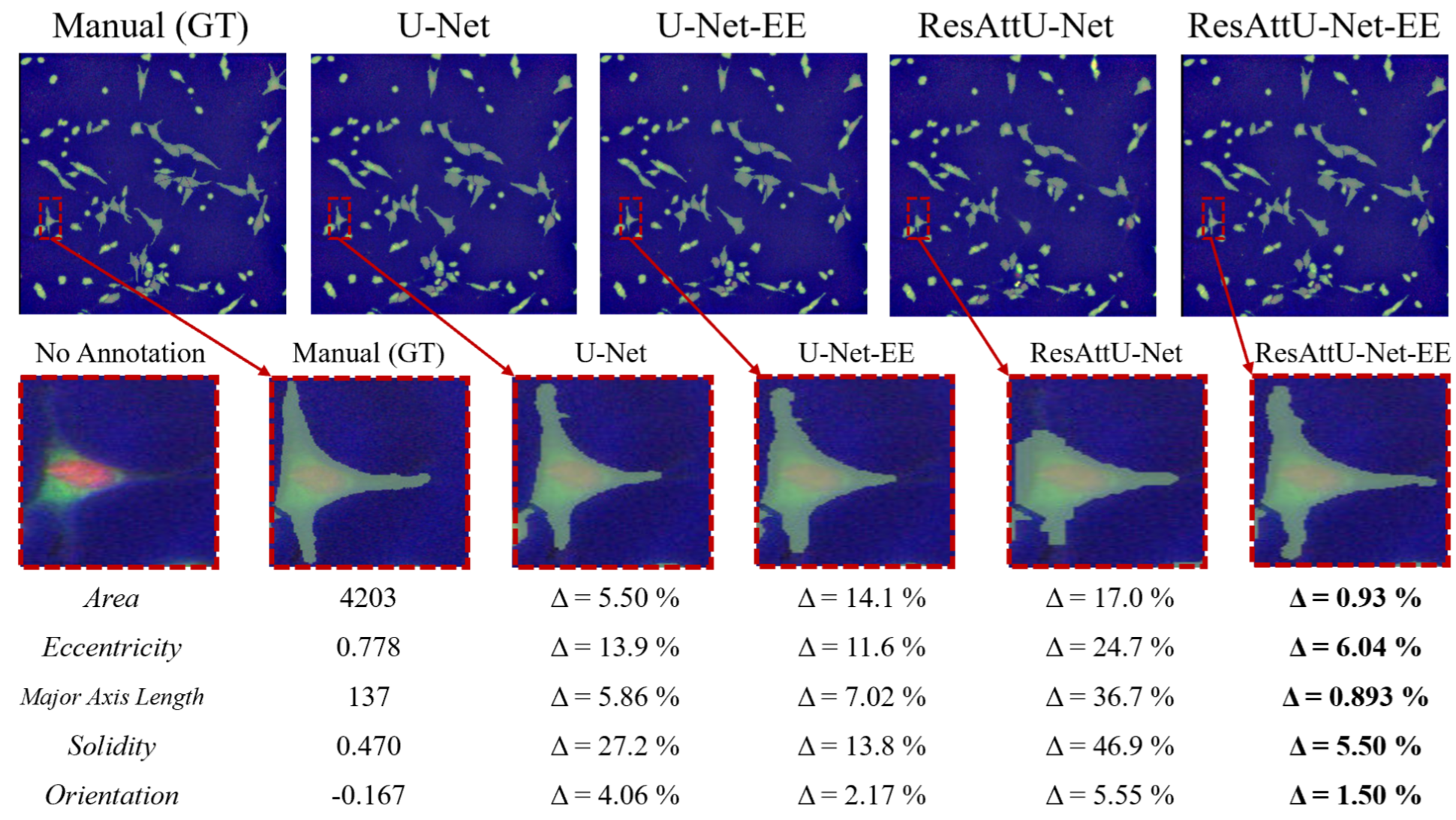
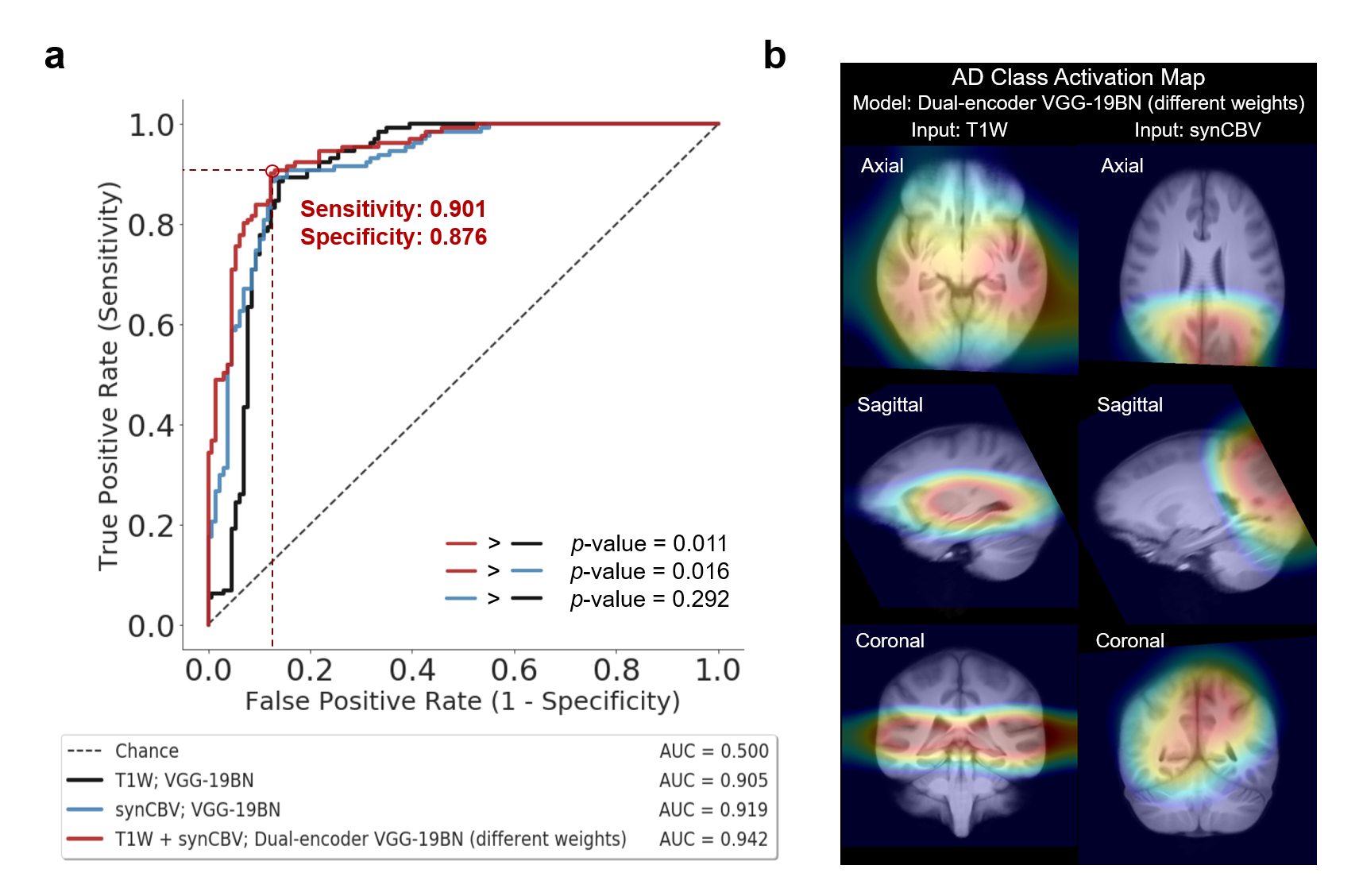
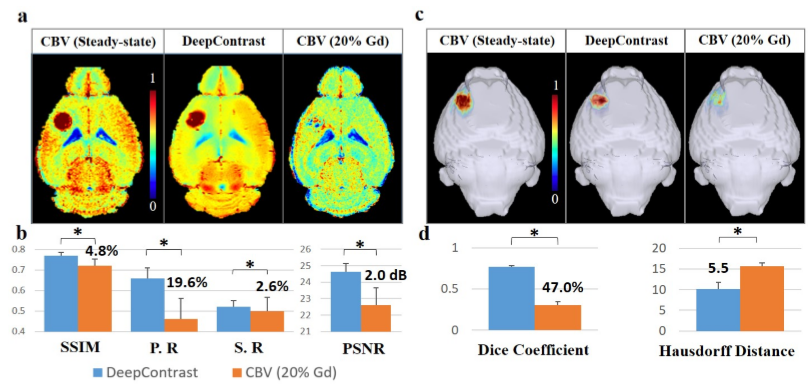
Substituting Gadolinium in Brain MRI Using DeepContrast
Haoran Sun, Xueqing Liu, Xinyang Feng, Chen Liu, Nanyan Zhu, Sabrina J Gjerswold-Selleck, Hong-Jian Wei, Pavan S Upadhyayula, Angeliki Mela, Cheng-Chia Wu, Peter D Canoll, Andrew F Laine, J Thomas Vaughan, Scott A Small, Jia Guo
IEEE ISBI 2020